By Ahmed El-Medany
Presented by Prof O’Regan, MRC Investigator and Consultant Radiologist, Imperial College London
The Digital Innovation in Cardiology session at BCS 2021 was a fascinating and inspiring insight into the technology available in various areas of Cardiology and covered exciting digital prospects for the future.
Prof O’Regan discussed Artificial Intelligence’s (AI) role in cardiovascular imaging; particularly when integrating imaging and non-imaging data, understanding new mechanisms of heart disease, and early diagnosis and risk prediction.
The speaker demonstrated some excellent examples of 3D segmentation cardiovascular MRI[1] (Figure 1).
‘Typically we rely on crude, quantitative measures of volumes and function’ Prog O’Regan stated. ‘A lot of this work is trying to make best use of the images of the data we already have’.
‘We can very readily and accurately scale imaging analysis to big cohorts and across different types of diseases…
We can also develop these very rich three-dimensional motion models of the heart, which allow us to capture the nature complexity of cardiovascular disease, that we may not be able to do with conventional measures’.
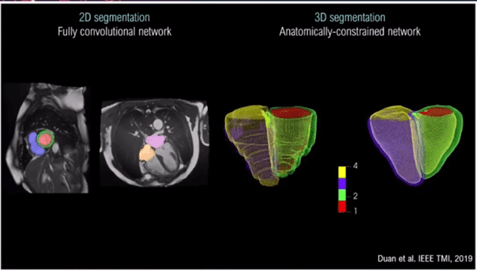
The presenter then showed an example of AI automated diagnosis based on 3D shape and motion, using a hypertrophic cardiomyopathy (HCM) model. ‘As clinicians looking at the image, we look at the whole image… we don’t just rely on the simple linear metric’.
An ‘auto-encoder’, a form of data compression, can be used to discriminate between different 3D heart models to reconstruct images specific to each cardiomyopathy (Figures 2 and 3). ‘[Automated diagnosis] captures, implicitly, differences in strain and regional differences in wall thickness’ the Professor stated. Moreover, this information can lead to us understating how the heart changes in shape and geometry, and the spectrum between health and disease [2].
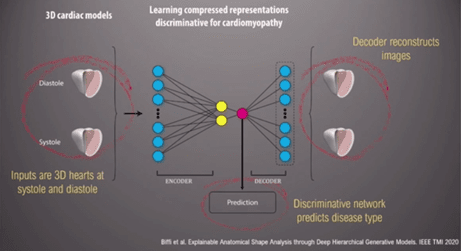
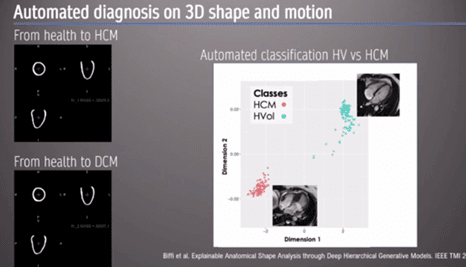
With regards to predicting outcomes in disease Prof O’Regan described a deep learning algorithm to automatically segment pulmonary hypertension MRI images, and predict survival based on motion of the right ventricle (RV) and interventricular septum (IVS).
Pixel precise analysis via AI and analysis of 300 points on the surface of the RV can create a digital model of how the RV moves in systole and diastole. Subsequently, aggregation of data from several hundred participants can be used to describe the variation in structure and function of the RV in a large cohort of patients.
Again, an auto-encoder can be used to find simple patterns in this complex data, useful for predicting survival in pulmonary hypertension (Figure 4) – a so-called ‘4DSurvival Network’ [3] .
Prof O’Regan stated: ‘Pulmonary hypertension outcomes at 1 year determine guideline driven management.. this can be really important for ensuring patients receive the right treatment’.
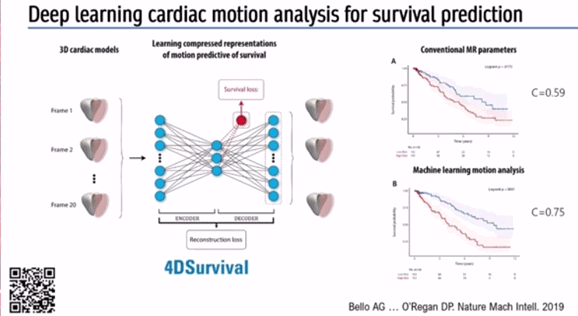
Next, the speaker discussed how imaging and genetics could be integrated using AI. 3D phenotype mapping (Figure 5) was performed in over 2,000 healthy individuals. Aggregated data was collected to look for truncating variants in Titin, a protein strongly implicated in dilated cardiomyopathy (DCM), which is also seen in around 1% of the healthy population[4,5].
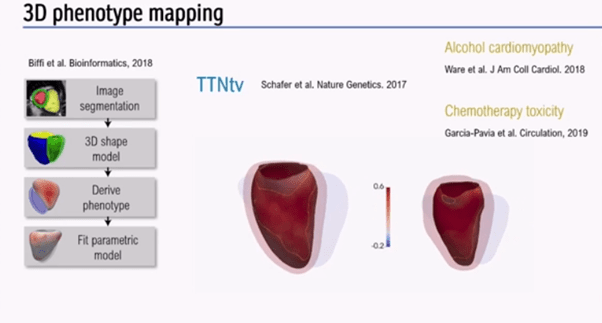
3D phenotype mapping (Figure 5) has shown that truncating variants have expressivity in healthy heart, although may not always lead to overt DCM, unless exposed to alcohol, chemotherapy, or pregnancy.
‘This can be a powerful way to integrate genetic data with imaging’ the presenter stated.
References
- DP, Rueckert D. Automatic 3D bi-ventricular segmentation of cardiac images by a shape-refined multi-task deep learning approach. IEEE transactions on medical imaging. 2019 Jan 23;38(9):2151-64.
- Biffi C, Cerrolaza JJ, Tarroni G, Bai W, De Marvao A, Oktay O, Ledig C, Le Folgoc L, Kamnitsas K, Doumou G, Duan J. Explainable anatomical shape analysis through deep hierarchical generative models. IEEE transactions on medical imaging. 2020 Jan 6;39(6):2088-99.
- Bello GA, Dawes TJ, Duan J, Biffi C, De Marvao A, Howard LS, Gibbs JS, Wilkins MR, Cook SA, Rueckert D, O’regan DP. Deep-learning cardiac motion analysis for human survival prediction. Nature machine intelligence. 2019 Feb;1(2):95-104.
- Biffi C, de Marvao A, Attard MI, Dawes TJ, Whiffin N, Bai W, Shi W, Francis C, Meyer H, Buchan R, Cook SA. Three-dimensional cardiovascular imaging-genetics: a mass univariate framework. Bioinformatics. 2018 Jan 1;34(1):97-103.
- Schafer S, De Marvao A, Adami E, Fiedler LR, Ng B, Khin E, Rackham OJ, Van Heesch S, Pua CJ, Kui M, Walsh R. Titin-truncating variants affect heart function in disease cohorts and the general population. Nature genetics. 2017 Jan;49(1):46-53.
Links
Full session available On Demand: https://bcs2021.co.uk
Deep-learning cardiac motion analysis for human survival prediction | Nature Machine Intelligence
Three-dimensional cardiovascular imaging-genetics: a mass univariate framework | Bioinformatics | Oxford Academic (oup.com)